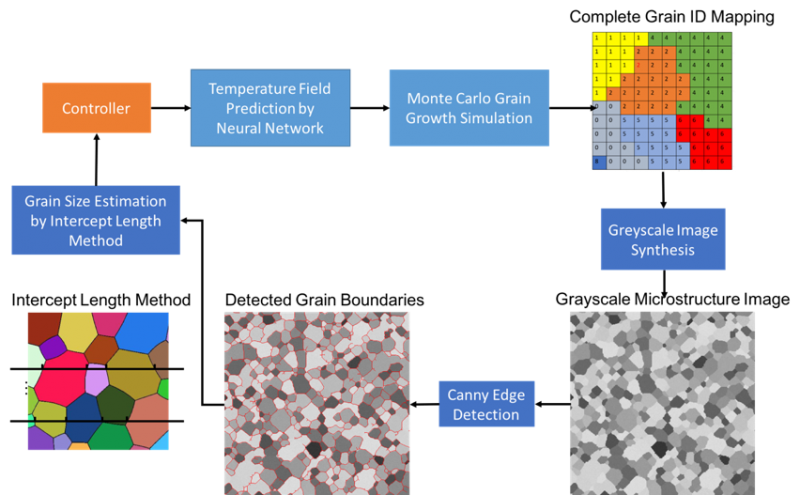
Distributed Heater Control of Grain Growth based on Image Feedback
Grain growth control involves a greater level of complexity, requiring the coupling of the temperature distributions from the FEM model to MC simulations of grain growth. The simulated grain sizes from each of ten zones associated with the spatial location of the underlying heater elements are used as the output feedback variables for the heater inputs. There is a multitude of challenges to this control problem: the model is nonlinear and stochastic, each heater input is coupled to the grain growth in multiple zones, and the input current is constrained by the maximum value. Our approach is to utilize the parabolic growth relationship, extended to the multiple zones above the heater elements. Three control strategies are considered: direct output feedback, inner-outer loop control, and model predictive control. Direct output feedback applies the consensus control architecture similar to the temperature control but uses the average grain size in each zone for feedback. The Passivity Theorem is used to show closed loop stability but feedback gains must be carefully tuned to avoid input saturation. Inner-outer loop control uses temperature as a surrogate input to more easily control the grain growth, and then adjusts the input current to achieve the required temperature. The challenge is that the heater input constraint does not easily translate to the temperature constraint. We have developed a real-time gain adjustment strategy to ensure that the surrogate temperature input is indeed achievable using the heater array. Model predictive control (MPC) solves a finite-horizon optimization to minimize the deviation of the grain growth from the desired trajectory subject to the input heater constraint. The optimization is repeated at regular intervals during runtime based on the measured averaged grain sizes. The inherent optimality and constraint handling is attractive, but the method demands high model fidelity and imposes higher online computation load. Evaluation of all three strategies with MC simulation shows convergence to the desired growth trajectory in all cases, but the inner-outer loop approach consistently gives the best performacne as it handles input constraint more efficiently than the direct output feedback case and is less prone to model stochasticity as in MPC. A key step in the closed loop grain growth control is the automatic measurement of the average grain size in each zone. We have applied image processing tools (edge detection, boundary gap closure, twin removal heuristics) to grain images to extract grain boundaries to automate this process. We have fully implemented the closed loop grain growth control based on the automated analysis of the synthetic grain images from the MC simulation coupled to the FEM thermal model, as depicted in the diagram below.